QWEN CHAT HUGGING FACE MODELSCOPE DASHSCOPE GITHUB PAPER DEMO DISCORD
We release Qwen2.5-Omni, the new flagship end-to-end multimodal model in the Qwen series. Designed for comprehensive multimodal perception, it seamlessly processes diverse inputs including text, images, audio, and video, while delivering real-time streaming responses through both text generation and natural speech synthesis. To try the latest model, feel free to visit Qwen Chat and choose Qwen2.5-Omni-7B. The model is now openly available on Hugging Face, ModelScope, DashScope,and GitHub, with technical documentation available in our Paper. Experience interactive capabilities through our Demo or join our Discord for discussions.
Key Features:
Omni and Novel Architecture: We propose Thinker-Talker architecture, an end-to-end multimodal model designed to perceive diverse modalities, including text, images, audio, and video, while simultaneously generating text and natural speech responses in a streaming manner. We prpose a novel position embedding, named TMRoPE (Time-aligned Multimodal RoPE), to synchronize the timestamps of video inputs with audio.
Real-Time Voice and Video Chat: Architecture Designed for fully real-time interactions, supporting chunked input and immediate output.
Natural and Robust Speech Generation: Surpassing many existing streaming and non-streaming alternatives, demonstrating superior robustness and naturalness in speech generation.
Strong Performance Across Modalities: Exhibiting exceptional performance across all modalities when benchmarked against similarly sized single-modality models. Qwen2.5-Omni outperforms the similarly sized Qwen2-Audio in audio capabilities and achieves comparable performance to Qwen2.5-VL-7B.
Excellent End-to-End Speech Instruction Following: Qwen2.5-Omni shows performance in end-to-end speech instruction following that rivals its effectiveness with text inputs, evidenced by benchmarks such as MMLU and GSM8K.
Architecture
Qwen2.5-Omni employs Thinker-Talker architecture. Thinker functions like a brain, responsible for processing and understanding inputs from text, audio and video modalities, generating high-level representations and corresponding text. Talker operates like a human mouth, taking in the high-level representations and text produced by the Thinker in a streaming manner, and outputting discrete tokens of speech fluidly. Thinker is a Transformer decoder, accompanied by encoders for audio and image that facilitate information extraction. In contrast, Talker is designed as a dual-track autoregressive Transformer Decoder architecture. During both training and inference, Talker directly receives high-dimensional representations from Thinker and shares all of Thinker’s historical context information. Consequently, the entire architecture operates as a cohesive single model, enabling end-to-end training and inference.
Performance
We conducted a comprehensive evaluation of Qwen2.5-Omni, which demonstrates strong performance across all modalities when compared to similarly sized single-modality models and closed-source models like Qwen2.5-VL-7B, Qwen2-Audio, and Gemini-1.5-pro. In tasks requiring the integration of multiple modalities, such as OmniBench, Qwen2.5-Omni achieves state-of-the-art performance. Furthermore, in single-modality tasks, it excels in areas including speech recognition (Common Voice), translation (CoVoST2), audio understanding (MMAU), image reasoning (MMMU, MMStar), video understanding (MVBench), and speech generation (Seed-tts-eval and subjective naturalness).
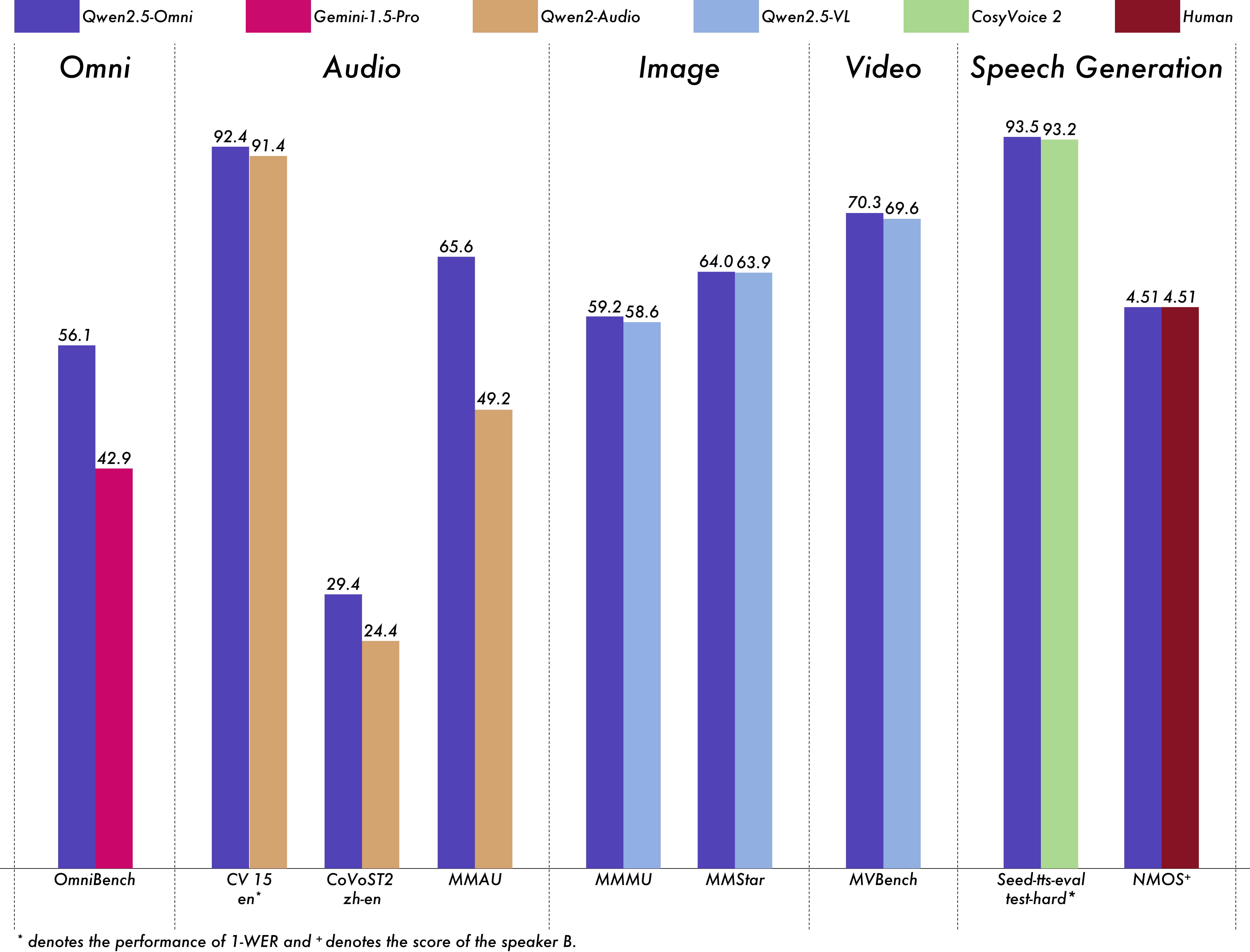
What’s Next
We are eager to hear your feedback and see the innovative applications you create with Qwen2.5-Omni. In the near future, our goal is to enhance our model’s ability to follow voice commands and improve audio-visual collaborative understanding. Additionally, we strive to integrate more modalities towards an omni-model!